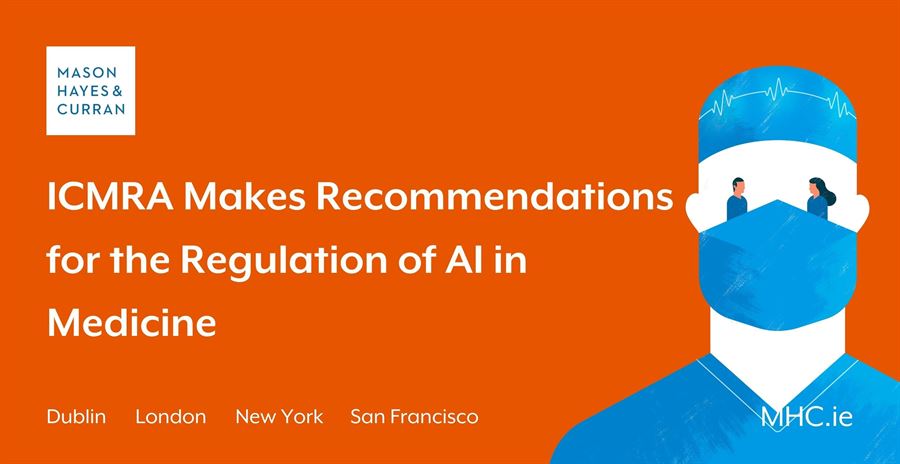
The International Coalition of Medicines Regulatory Authorities (ICMRA) published a report in August 2021 on Artificial Intelligence (AI). This Report sets out a number of recommendations to assist regulators in addressing the challenges that this technology poses to the regulation of global medicines. Preclinical development, clinical trial data recording and analysis, pharmacovigilance and clinical use optimization are just a few examples of how AI technologies are being deployed across all stages of a medicine’s lifecycle. However, while AI has undoubtedly strengthened the rate of innovation in healthcare and medicine, its unique characteristics and rapid development present new challenges to the existing regulatory framework.
We discuss some of the regulatory challenges posed by the use of AI in the development of medicines. This will set the stage for an examination of the key findings and recommendations set out in the ICMRA’s report. The implementation of the Report will be discussed by ICMRA members in the coming months.
Key Challenges for AI in Medicine
AI holds enormous potential for strengthening the delivery of medicine by facilitating the rapid development of new and innovative drugs. However, its ever-increasing use in healthcare coupled with its unpredictability and rapid development presents unique challenges for manufacturers and regulators including:
-
Development: Many AI technologies deployed in a medicinal product’s lifecycle are regulated under the Medical Device Regulation (EU) No 2017/745 (MDR) as software medical devices. The MDR requires software devices to be designed in a manner that ensures repeatability, reliability, and performance in line with their intended use. However, AI models, particularly in the field of machine learning, are trained and tested using large data sets with conventional software algorithms, which makes them difficult to validate and verify using existing standards.
-
Transparency: AI machine learning systems that deploy deep learning tools, criticised as “black boxes”, will perform billions of calculations to arrive at a decision or result. This complexity makes it very difficult to trace or diagnose the source of incorrect results in a meaningful way, which in turn gives rise to further validation and verification challenges for regulators.
-
Data privacy: AI systems deployed in a healthcare context engage in ‘automated processing’ of personal data, need to comply with the EU’s General Data Protection Regulation (EU) No 2016/679 (GDPR). This is required by the MDR, and specifically identified as an issue by the Report as it poses several challenges for manufacturers. For example, where AI software is used in the clinical trial of a new drug, manufacturers would need to provide patients with ‘meaningful information about the logic involved’ in any automated decision taken by an AI algorithm relating to their care. In addition, manufacturers need to ensure they have a lawful basis for processing. Where health data is being processed this often means obtaining informed consent from the patient.
-
Data and bias: Many deep learning AI models are trained on very large data sets. Successfully ensuring that the quality and integrity of this training data is maintained is a highly complex, time-intensive, and costly process. Apart from the integrity of this data, statistical distribution of data must be carefully managed to maintain algorithmic hygiene. Training AI with data that is not representative of the actual environment that the model is designed to operate in can lead to results that will reflect the biases in the data. This in turn can trigger moral and ethical questions as well as challenges affecting the safety and performance of the AI in the development and use of medicinal products.
The ICMRA Report
The ICMRA Report details the outcome of a horizon scanning exercise performed by the ICMRA’s Informal Network for Innovation working group. This group comprised of ICMRA member regulators from Italy, Denmark, Canada, Ireland, Switzerland, the World Health Organisation and the European Medicines Agency (as working group lead). The horizon scanning process is used to develop hypothetical case studies to stress-test the existing regulatory frameworks of the ICMRA members and develop recommendations to adapt them to the challenges and issues identified.
The two case studies developed by the working group for this report were:
- A central nervous system app supported by AI. This could record and analyse baseline disease status for the selection of patients for clinical trials, as well as monitor adherence and response to therapies to measure efficacy and effectiveness, and
- The use of AI in pharmacovigilance, the process of monitoring the safety of medicines, in terms of the detection of safety signals by reducing the heavy manual component of current tools and enabling the discovery of safety signals that are difficult to detect with current methods.
Considering the case studies, the Report makes a number of recommendations for regulators and stakeholders involved in medicine development to foster the uptake of the technology. Some of the key recommendations include:
-
Establishing a permanent ICMRA working group, or a standing ICMRA agenda item on AI to allow member agencies to share their experiences of regulating AI and best practices for its use.
-
Regulators may need to implement a risk-based approach to assessing and regulating AI, which could be informed through collaboration in the ICMRA. Legal and regulatory frameworks may need to be adapted to facilitate scientific or clinical validation of AI which requires a sufficient level of understandability and regulatory access.
-
Sponsors, developers, and pharmaceutical companies should establish strengthened governance structures to oversee AI algorithms and deployments that are closely linked to the benefit/risk of a medicinal product. This would involve establishing a multi-disciplinary oversight committee for product development to understand and manage the implications of higher-risk AI.
-
Regulators should consider establishing the concept of a Qualified Person responsible for AI and/or algorithm(s) oversight compliance.
-
Regulatory guidelines for AI development and use with medicinal products should be developed in areas like data provenance, reliability, transparency and understandability, validity, pharmacovigilance, and real-world performance monitoring of patient functioning, and
-
The EU post-authorisation management of medicines may need to be adapted to accommodate updates to AI software linked to a medicinal product.
Conclusion
The rapidly growing use and evolution of AI in the development of medicines has brought with it a myriad of ethical and regulatory challenges. Regulators need to consider how they can foster the uptake of this technology while at the same time protecting the health and safety of consumers. While the European Commission has recently published its Proposal for a Regulation on AI setting out plans for a comprehensive regulatory framework for the technology, it remains the case that no international guidance, common specifications and/or harmonised standards currently exist for the use of AI tools in the development of medicines. The ICMRA’s recommendation for the establishment of regulatory guidelines for the use of AI in this space is thus a welcome development.
For more information, contact David Culleton, or a member of our Life Sciences team.
The content of this article is provided for information purposes only and does not constitute legal or other advice.