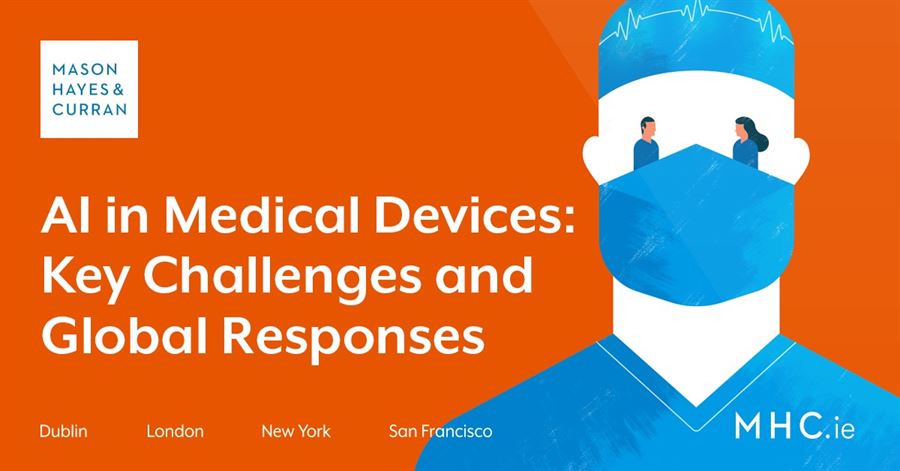
The European Commission published its Proposal for a Regulation on Artificial Intelligence (AI) in April of this year. The Proposal set out plans for a comprehensive regulatory framework that has the potential to set a global standard for the regulation of AI. Although elements of this proposal address high-risk AI applications, which are directly relevant to the MedTech sector, it remains the case that no international guidance, common specifications and/or harmonised standards currently exist for the use of AI tools in medical devices. As the availability of medical devices that use sophisticated AI continues to increase however, regulators are developing new policies and guidance that can ensure that the power and promise of these new technologies can be harnessed and utilised safely and reliably.
Key challenges for AI in medical devices
In an increasingly dynamic and innovative market for digital health solutions, the development and use of medical device software in healthcare has already served to challenge and disrupt existing regulatory frameworks.
In addition, regulators are now being called on to grapple with questions of how medical device software is to be regulated when AI algorithms also form a part of its programming.
This requires an understanding of some of the unique challenges that AI can give rise to in the context of healthcare:
- Development: The Medical Device Regulation (MDR) requires software devices to be designed in a manner that ensures repeatability, reliability and performance in line with their intended use. However, these types of requirements are more easily satisfied when developing and programming conventional software algorithms as opposed to AI. This is because AI models are rarely programmed ‘line by line’. Instead, many AI applications, particularly in the field of machine learning, are trained and tested using large data sets. This approach makes them difficult to validate and verify using existing standards.
- Transparency: A related challenge that is particularly significant for machine learning algorithms based on neural networks is the so called ‘black box’ issue. A sophisticated multilayer neural network will perform billions of calculations in order to arrive at a decision or result. This complexity makes it very difficult to trace or diagnose the source of incorrect results in a meaningful way. Unfortunately, this gives rise to further challenges in terms of validation and verification.
- Instability: A lack of clarity as to exactly how some AI algorithms, especially those in the field of machine learning, are processing input data in order to produce defined outcomes has given rise to a growing awareness around ‘adversarial’ learning. This lack of clarity also gives rise to the potential for deceptive input to be introduced to an AI model with the intention of destabilising the model and causing it to generate incorrect outputs. This issue has been illustrated in several studies involving visual AI tools. The studies showed how distortions to images that are barely visible to the human eye can trigger major errors by the AI system being targeted by the adversarial attack. Although these types of challenges are particularly serious in terms of the safety and performance of medical devices, ‘adversarial training’ techniques and ‘human-in-the-loop’ mechanisms that protect algorithms are being developed to make AI algorithms more resilient to these types of vulnerabilities.
- Data privacy: AI systems deployed in a healthcare context, which engage in ‘automated processing’ of personal data, need to comply with the GDPR. This is specifically identified as an issue by the MDR. This compliance creates a number of challenges for manufacturers. For example, manufacturers need to provide patients with ‘meaningful information about the logic involved’ in any automated decision taken by an AI algorithm relating to their care. This can be challenging where these decisions rely on sophisticated and dynamic AI models. Manufacturers also need to ensure they have a lawful basis for processing, and where health data is being processed this often means obtaining informed consent. As the training of complex neural networks involves preparation of very large training/ testing sets of data and sophisticated and dynamic processing that may change over time, manufacturers will need to ensure they have identified and explained all the ways in which they want to use the data to the patient at the outset in order to rely on that consent.
- Data and bias: Many deep learning AI models are trained on very large data sets that must be carefully identified and painstakingly labelled before being used. Successfully ensuring the quality and integrity of this training data is maintained is a highly involved process. The time and cost involved in preparing these data sets, something that has traditionally been done by human processors, has an important relationship with the performance of the model. Apart from the integrity of this data, statistical distribution of data must also be carefully managed. Training AI with data that is not representative of the actual environment that the model is designed to operate in can also lead to results that will reflect the biases in the data. This in turn creates a potential problem that triggers moral and ethical questions as well as challenges affecting the safety and performance of AI as a medical device.
Emerging guidance
Guidance specifically on AI has not yet been published by the EU Medical Device Coordination Group (MDCG). Guidance, however, for AI under the MDR/IVDR framework has been assigned to the Borderline & Classification (B&C) Working Group, but no timeline has been set out for delivery.
Regulators in various jurisdictions beyond the borders of the EU have also been developing proposals and guidance designed to tackle these emerging challenges over the last number of years, for example:
• The South Korean Ministry of Food and Drug Safety (MFDS) has released multiple guidance documents related to software using AI, Big Data and Machine Learning. In June 2017, the MFDS also published the world’s first AI medical device permit and examination system and was elected as the first Chair of the International Medical Device Regulators Forum (IMDRF) Artificial Intelligence Medical Devices (AIMDs) working group in June 2020.
• The US Food and Drug Administration (FDA) published a discussion paper in 2019 entitled Proposed Regulatory Framework for Modifications to Artificial Intelligence/Machine Learning-Based Software as a Medical Device. The paper proposed a framework based on the internationally harmonised IMDRF risk categorisation principles for software medical devices, FDA’s benefit-risk framework, the organisation-based Total Product Life Cycle approach as envisioned in the FDA Digital Health Software Precertification Program, as well as practices from existing FDA premarket programs such as the 510(k), De Novo, and Premarket approval pathways. More recently, the FDA published its action plan on furthering AI in medical devices in January 2021.
• Most recently on 28 June 2021, the World Health Organization also published Ethics & Governance of Artificial Intelligence for Health which sets out six consensus ethical principles for the appropriate use of AI for health.
These documents serve as a useful starting point when moving to piece together an emerging international patchwork of regulatory guidance in this area.
Conclusion
Research and development of AI applications for the healthcare sector continues to gather pace internationally. In tandem, more and more medical devices incorporating AI are arriving to market. As a result, there is a pressing need for a coherent, consistent and ultimately harmonised approach to the regulation of these powerful yet poorly understood technologies. Work to develop the required regulatory guidance is well underway and interested stakeholders should actively monitor developments in the various regions and jurisdictions in which they operate in order to stay abreast of this continuously evolving regulatory landscape.
It is hoped that growing awareness around the risks and challenges presented by the use of AI in healthcare, as well as the policies being developed to manage those risks, can also continue to foster increased credibility and public trust in these technologies, which are set to shape the provision of healthcare for years to come.
For more information, contact a member of our Life Sciences team.
The content of this article is provided for information purposes only and does not constitute legal or other advice.
Share this: